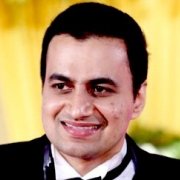
Tejas Dattatraya Kulkarni is a PhD student in the Department of Brain and Cognitive Sciences and Computer Science and Artificial Intelligence Laboratory at the Massachusetts Institute of Technology. He received his Bachelor’s in Electrical and Computer Engineering from Purdue University in 2010. His broad research interest is at the intersection of Artificial Intelligence and Cognitive Science. He is specifically interested in bridging probabilistic graphical models, probabilistic programming and fast discriminative methods. Recently, he has been focusing on applying such techniques in the domain of vision as inverse graphics. He has been awarded the Henry Singleton award and the Leventhal Fellowship for his graduate work. In January 2015, Tejas was named as the first CBMM Siemens Graduate Fellow.
Research Statement:
One of the central goals of computational vision is to get symbolic description of scenes from natural images. Vision as inverse graphics is one of the most elegant formulations that could take us closer towards this goal. In the past, because of computational limitations, it has been hard to directly implement this approach in practice. However, it may be right time to revisit this idea due to significant advances in computer graphics, computational statistics and computer vision.
Probabilistic programming allows us to express complex models while keeping the inference machinery general-purpose.Formalizing inverse graphics as probabilistic programming could allow us to solve even more complicated vision problems with general purpose machinery. In order to scale this approach, our research incorporates techniques such as: data-driven proposal learning, deep learning, approximate bayesian computation, differentiable rendering and exploiting conditional independences in the rendering pipelines. Computer Graphics seems to be advancing at a great pace in terms of designing solutions for hard image synthesis problems. The combination of generative modeling, approximate inference, computer graphics and deep learning could utilize such advances directly to solve the hard inversion problem.